What Are The Difference Between ChatGPT Vs. Bard Vs. Bing?
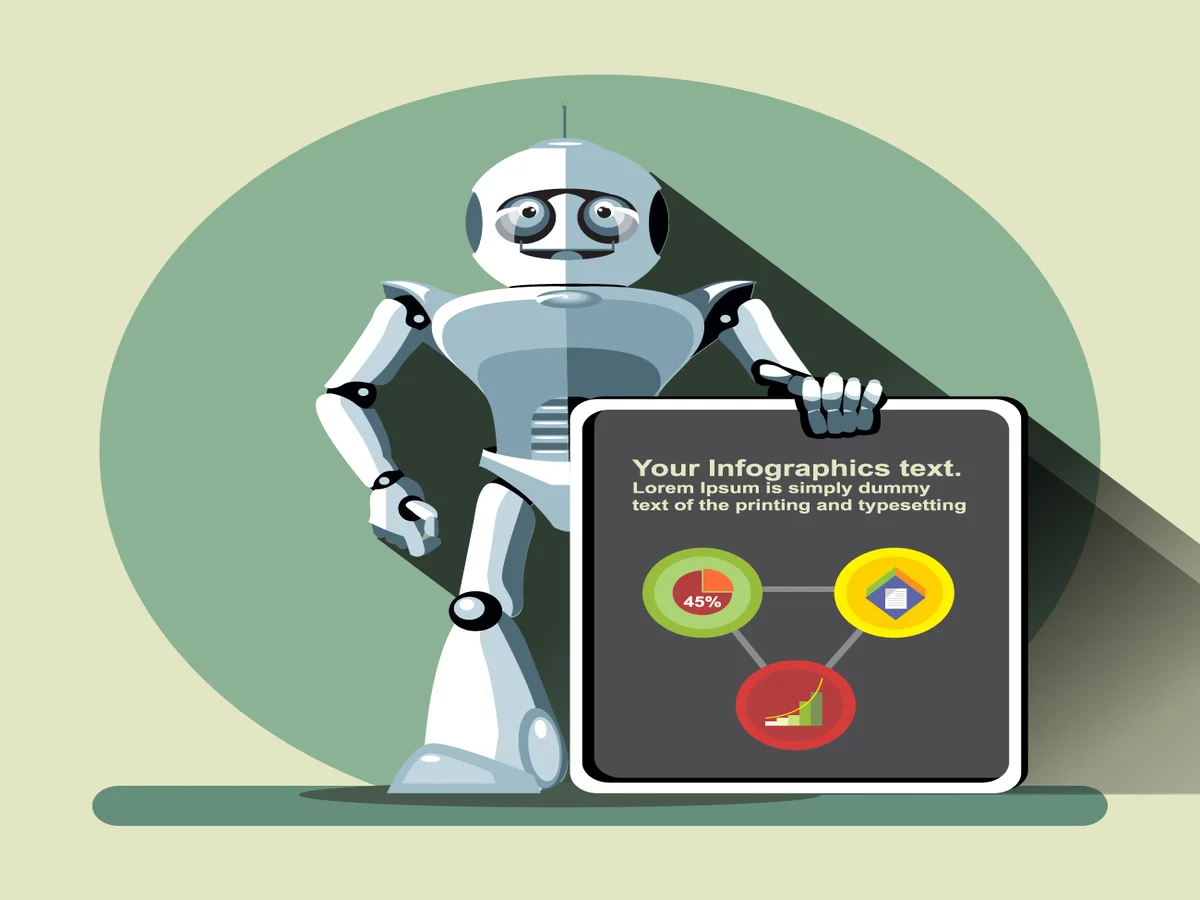
In the realm of natural language processing (NLP) and conversational AI, several powerful models have emerged, each with its unique characteristics and applications. Among them, ChatGPT, BERT (Bidirectional Encoder Representations from Transformers), and Bing stand out as notable players in the field. Let’s delve into the key differences between these three and understand their respective strengths and weaknesses.
1. Architecture:
ChatGPT:
ChatGPT is based on the GPT-3.5 architecture, developed by OpenAI. It belongs to the family of transformers, specifically designed for handling sequential data and excelling in generating human-like text. GPT-3.5 is known for its large-scale training data and impressive language understanding capabilities.
BERT:
BERT, on the other hand, is a transformer-based model developed by Google. Unlike traditional models that process words in order, BERT employs a bidirectional approach, considering the entire context of a word in a sentence. This bidirectionality enables BERT to capture more nuanced relationships between words.
Bing:
Bing, in this context, refers to the search engine developed by Microsoft. While not a language model like ChatGPT or BERT, Bing is a search engine that utilizes various algorithms, including natural language processing, to retrieve relevant information from the web.
2. Training Approach:
ChatGPT:
ChatGPT is trained using a method called unsupervised learning on a massive dataset. It learns from diverse internet text, absorbing patterns, context, and language nuances. The training process involves predicting the next word in a sentence, allowing the model to grasp the intricacies of language.
BERT:
BERT employs a pre-training and fine-tuning strategy. In the pre-training phase, the model learns from vast amounts of text data, understanding the relationships between words. Fine-tuning is then performed on specific tasks, making BERT adaptable to various NLP applications.
Bing:
Bing’s algorithms are trained using a combination of supervised and unsupervised learning. The search engine learns from user interactions, clicks, and explicit feedback to improve its ranking and relevance in providing search results.
3. Use Cases:
ChatGPT:
ChatGPT is designed for natural language understanding and generation. Its applications range from chatbots and virtual assistants to content creation and code generation. Its strength lies in producing coherent and contextually relevant responses in a conversational context.
BERT:
BERT excels in tasks requiring a deep understanding of context, such as question-answering and sentiment analysis. It has proven effective in various NLP benchmarks and competitions, showcasing its versatility in different applications.
Bing:
Bing serves as a search engine, assisting users in finding information on the internet. Its applications extend beyond text to include image and video search, providing a comprehensive search experience.
4. Scale and Size:
ChatGPT:
ChatGPT, specifically GPT-3.5, is renowned for its massive scale, with 175 billion parameters. This extensive size contributes to its ability to understand context, generate coherent responses, and perform a wide range of language-related tasks.
BERT:
While not as large as GPT-3.5, BERT is still a sizable model, typically consisting of hundreds of millions of parameters. Its size contributes to its ability to capture intricate language patterns during pre-training.
Bing:
Bing’s algorithms operate on a vast index of web pages, providing users with a comprehensive search experience. The scale of Bing’s database and the efficiency of its ranking algorithms contribute to its effectiveness as a search engine.
5. Limitations:
ChatGPT:
One limitation of ChatGPT is its occasional generation of responses that may be contextually incorrect or nonsensical. It can also be sensitive to input phrasing and may produce different responses with slight rephrasing.
BERT:
While powerful, BERT may struggle with handling long documents due to its token-based approach. It may also require substantial computational resources for training and inference.
Bing:
Bing’s limitations lie in its dependence on available web content. It may not always provide the most up-to-date or accurate information, and its search results can be influenced by the popularity of content rather than its relevance.
ChatGPT, BERT, and Bing serve distinct purposes in the field of natural language processing. ChatGPT excels in generating human-like text and facilitating conversations, BERT is proficient in understanding context for various tasks, and Bing operates as a search engine providing access to information across the web. Understanding these differences is crucial for selecting the right tool or model based on specific application requirements.
FAQs:
ChatGPT is designed for natural language understanding and generation. It finds applications in chatbots, virtual assistants, content creation, and code generation.
ChatGPT is trained using unsupervised learning on a massive dataset. It learns from diverse internet text by predicting the next word in a sentence, enabling it to understand language patterns and context.
ChatGPT is based on the GPT-3.5 architecture, a transformer model with 175 billion parameters. This architecture enables it to handle sequential data and generate coherent and contextually relevant responses.
BERT employs a bidirectional approach, considering the entire context of a word in a sentence. This bi-directionality allows BERT to capture more nuanced relationships between words, enhancing its understanding of context.
Bing is a search engine developed by Microsoft. It enables users to search for information on the internet, including web pages, images, and videos.